Haab
Laboratory
Pancreatic Cancer Identification Using Glycans
Pancreatic ductal adenocarcinoma (PDAC) is the single most aggressive and lethal form of cancer yet described. Recent estimates suggest that 64,050 individuals will be newly diagnosed with PDAC, and another 50,550 will die from the disease in 2023 in the United States alone. Five-year survival of people diagnosed with PDAC is 11%. Notably, people whose PDAC was identified at a resectable stage and who underwent surgical resection had an almost four-fold better five-year survival rate (up to 44%) compared with patients with unresectable PDAC (12%). However, early-stage PDAC is generally asymptomatic and only ~15% of people are diagnosed sufficiently early for surgical resection to be a viable treatment option. Therefore, the long-term goal of the Haab Laboratory is to reduce the burden of PDAC in the U.S. population by enabling early disease detection to improve treatment outcomes.
PDAC is a relatively rare cancer. Magnetic resonance imaging or endoscopic ultrasound is currently required for a definitive disease diagnosis. However, routine imaging of the general population is not feasible. Therefore, a convenient, low-cost method, such as a blood test, could solve this problem and could make surveillance both practical and economically feasible. A significant challenge in disease diagnosis is that pancreatic cancers are different from other types of cancer in that there can be multiple cancer cell lineages contained within a single tumor, each with its own characteristics including propensity to metastasize, response to treatments and production of molecular markers. Because of this heterogeneity, no single method of detection or treatment works equally well over all pancreatic cancers.
As a solution to this challenge, the Haab Laboratory has recently identified a combination of blood biomarkers that identify two main subtypes of PDAC cancer cells that make up a tumor. These biomarkers, which are specific types of carbohydrate known as glycans, appear on the surfaces of PDAC tumor cells and are shed into the blood circulation. The detection of altered cancer cell specific glycans in the blood circulation using blood tests developed by the Haab Laboratory have significantly improved upon the current best methods for PDAC identification.
Building upon these advances, the Haab Laboratory is now focused on defining the glycans that are uniquely characteristic to each PDAC cancer cell subpopulation beyond the two main types we have previously identified in order to develop further simple blood tests that will enable the earlier detection and treatment of this aggressive and highly lethal form of cancer. In addition, by characterizing the biological and functional differences between the subpopulations — in particular the metabolic underpinnings of each of the subpopulations, the laboratory is further developing the tests to guide treatment decisions based on the subpopulations and their predicted responses to treatments.
Our Impact
We’re raising thousands to save millions.
We’re turning hope into action for the millions of people around the world affected by diseases like cancer and Parkinson’s. Find out how you can help us make a difference.
- 121 peer-reviewed papers published in 2023
- 62 peer-reviewed papers published in high-impact journals in 2023
- 55 clinical trials launched to date
Brian Haab, Ph.D.
Professor, Department of Cell Biology
Areas of Expertise
Pancreatic cancer, glycobiology, biomarker development, technology development, microarray methods
Biography
Dr. Haab obtained his Ph.D. in chemistry from the University of California at Berkeley in 1998. He then served as a postdoctoral fellow in the laboratory of Patrick Brown in the Department of Biochemistry at Stanford University. Dr. Haab joined VAI as a Special Program Investigator in May 2000, became a Scientific Investigator in 2004, and was promoted to Senior Scientific Investigator in 2007 and promoted to Professor in 2013. He recently served as Assistant Dean of Van Andel Institute Graduate School and as an adjunct professor in the Department of Biochemistry and Molecular Biology and the Genetics Program at Michigan State University.
Cancer cell production of atypical combinations of glycans to distinguish cancer subpopulations
Although a subset of PDAC patients respond to chemotherapy, leading to extended survival, another subset of patients does not. Even among patients with localized disease who receive surgery and aggressive chemotherapy, about 40% experience disease relapse within 1.5 years and about 30% survive <2 years past surgery. Identifying this poor-prognosis subset prior to treatment is currently not possible, as the prognostic accuracy of conventional measures is insufficient and diagnostic and prognostic molecular biomarkers are lacking. As a result, many patients who will not benefit from chemotherapy are unnecessarily exposed to harmful side effects. At the same time, others go undiagnosed until treatment options have narrowed. In addition, it is currently not possible to design clinical trials that can test for differential responses between the short-survival and extended-survival cohorts because the cohorts cannot be distinguished prior to treatment.
One of the drivers of PDAC progression is heterogeneity in the cancer cells within a tumor. Gene-expression profiling studies on whole-tumor mRNA have uncovered two main PDAC cell subtypes: classical and basal (or squamous). Using microdissection, single-cell sequencing, and spatial transcriptomics, we have demonstrated that PDAC tumors comprise an admixture of the two main subtypes, along with other, less-well-characterized PDAC cancer cell subtypes. Such cancer cell subtype heterogeneity — which can develop through outgrowths from progenitor populations, changes in the microenvironment, or alterations in epigenetic drivers — is one of the likely reasons that PDAC is so relentlessly aggressive and lethal. Therefore, the measurement of intratumoral heterogeneity in cancer cell subpopulations offers a new strategy to identify poor-prognosis patients prior to treatment. However, the exploration of intratumoral heterogeneity as a quantifiable biomarker of prognosis has been limited, in part owing to the lack of practical and precise biomarkers for cancer-cell subpopulations.
Our previous work has shown that combinations of glycan biomarkers secreted from the cell surface can distinguish between different PDAC cancer cell subtypes and can be used to quantify intratumoral heterogeneity. It is the pattern, or signature, of glycans that form specific markers and that enable high specificity recognition. By immunofluorescent tumor imaging, we have observed that only certain subpopulations of cancer cells produce a glycan called CA19-9, while the glycan biomarker STRA is produced by a different subpopulation of cancer cells. Some cancer cell subpopulations within tumor samples produce only CA19-9, some produce only STRA, some produce both, and some produce neither. We have now identified additional glycans that, in combination with CA19-9 and STRA, identify additional PDAC cancer cell subpopulations. Such biomarkers form a novel approach to distinguish between PDAC cancer cell subtypes and to quantify intratumoral heterogeneity. Therefore, our ongoing work is focused on identifying more PDAC cancer cell subpopulations so that we can determine the functional differences between the glycan-defined subpopulations that will enable earlier disease detection and the development of targeted treatment options.
Enabling clinical use: high-sensitivity serum assays of subpopulation-specific glycan combinations
For the findings described above to have the greatest possible impact on improving patient outcomes, the biomarkers should be applicable in a low-cost and convenient test, such as a blood serum assay. An advantage of glycan signatures is the potential for detecting the glycans in blood serum. We previously showed a direct correlation between the tumor glycan levels and the serum glycan levels for STRA and CA19-9. These results show that, for certain glycans, the blood levels are a good indicator of the tumor levels. Other glycans produced on PDAC cells are known to be secreted into the blood, such as sialyl Lewis X. As such, we are investigating the correlation between the observed glycan signatures in tumor tissue and observable serum biomarkers and developing the serum assays that are indicative of the tumor levels of specific glycan-defined cancer subpopulations.
While the standard CA19-9 assay captures and detects the CA19-9 antigen, CA199.STRA is a “hybrid” glycan sandwich assay, in which the capture antibody binds to CA19-9 and the detection antibody binds STRA. In several rigorously controlled blinded studies, using a combination of the CA199.STRA sandwich assay and the standard CA19-9 assay significantly improved the ability to detect PDAC over the CA19-9 test alone. In each study, approximately half of the patients who were not positive for CA19-9 were positive for CA199.STRA, allowing their PDACs to be identified earlier than possible with CA19-9 alone. This performance meets targets needed for further development as a clinical diagnostic biomarker.
Additional serum assays are now under development for the various PDAC cancer cell subpopulations being defined in our studies of tumor tissue. Each of these subpopulations is defined by a combination of glycans that can be detected using the hybrid glycan sandwich method. The laboratory is now working to validate each serum assays as a reliable indicator of the presence of a specific cancer subpopulation in the tumor. The integrated use of the serum assays for the distinct cancer subpopulations promises to provide an effecting way to detect pancreatic cancer and to guide treatment according to guide treatment.
Glycan synthesis in cancer cells as an indicator of metabolic phenotype
What causes the glycan differences between cells, and in particular, the glycan differences between PDAC cancer cell subpopulations? Monosaccharides — particularly glucose — are converted to glycan precursors and also are used for energy generation The regulation of glycan synthesis therefore inherently connects to cellular metabolism. Cancer cells frequently increase their reliance on glucose for energy, but the resulting effects on glycan production are not clear. We hypothesized that shifts in the use of glucose for energy alter the availability of glucose for conversion to nucleotide-sugar precursors and subsequent synthesis of extracellular glycans.
Through understanding this relationship, we plan to use detection of the glycans to identify the metabolic abnormalities of the cancer cells, which, in turn, could be used to select treatment methods optimized to the cancer’s metabolism. As an example of such treatment, metformin, the widely used diabetes drug, shows efficacy in vitro against pancreatic cancer cells of one metabolic type but not against all PDAC cancer cell subtypes. Our research is defining the glycans that are produced uniquely by the cancer cells that are responsive to metformin.
To enable such studies, we have developed methods to track isotope-labeled glucose carbons in the media on their journey through nucleotide-sugar precursors to mature membrane-bound glycans. We also have developed a method to standardize monosaccharide abundance thereby enabling comparisons between cell lines with widely varying metabolic characteristics or growth conditions. Our work has demonstrated that the commitment of glucose to energy production determines the glucose available for nucleotide-sugar precursor synthesis and glycan production. Cancer-cells that are adapted to glucose for energy correspondingly limit their extracellular glycosylation, suggesting that the extracellular glycosylation of cancer cells is fundamentally modulated by the type of metabolic adaptation. Ongoing work is devoted to mapping the effects of cancer-associated glycans and identifying the specific glycan features that can be used for metabolic classification and prediction of treatment responses.
CarboGrove
CarboGrove is a database of the binding specificities of glycan-binding proteins. The results are derived from MotifFinder analysis of glycan array data from a wide variety of glycan array sources. In addition to presenting detailed summaries of lectin and antibody binding, the database offers programmatic access to the data through an API as well as a web server for running MotifFinder.
Visit CarboGroveMotifFinder
MotifFinder enables the automated analysis of glycan array data. The program provides information about the binding determinants of lectins and glycosidases that were incubated on the arrays. Full descriptions and instructions are provided in the download material.
MotifFinder Request Form
Please fill out the form below to request the MotifFinder software.
MotifFinder Request Form
"*" indicates required fields
SignalFinder-Microarray
SignalFinder-Microarray (SignalFinderMA) enables the automated analysis of microarray images. SignalFinderMA automatically detects individual spots within a microarray and outputs statistics for each spot and for replicate spots. The program can accommodate multiple arrays within a single image. Full descriptions and instructions are provided in the download material.
SignalFinder-Microarray Request Form
Please fill out the form below to request the SignalFinder-Microarray software.
SignalFinder-Microarray
"*" indicates required fields
SignalFinderIF
SignalFinderIF is the core of the SignalFinder Suite. SignalFInderIF enables the automated analysis of pathology tissues stained with immunofluorescence and imaged with a microscope or digital pathology platform. SignalFinderIF implements the Segment Fit Thresholding (SFT) algorithm to determine and quantify signal objectively from immunofluorescence images. The software provides the ability to select as many channels as are available from the image file as well as multiple thresholds selected as multipliers of the base threshold with user selection of channels, thresholds and multiplier. SignalFinder also allows selection of multiple tissues and provides automated tissue detection for tissue selection from tissue microarrays (TMAs). The software supports multiple image formats and can be quickly adapted to most image formats, upon request.
SignalFinderIF Request Form
Please fill out the form below to request the SignalFinderIF software.
SignalFinderIF
"*" indicates required fields
SELECTED PUBLICATIONS
For a full list of Dr. Haab’s publications, please visit PubMed.
2023
Wisniewski L, Braak S, Klamer Z, Goa CF, Shi C, Allen P, Haab BB. 2023. Heterogeneity of glycan biomarker clusters as an indicator of recurrence in pancreatic cancer. Front Oncol 13.
2022
Klamer ZL, Harris CM, Beirne JM, Kelly JE, Zhang J, Haab BB. 2022. CarboGrove: a resource of glycan-binding specificities through analyzed glycan-array datasets from all platforms. Glycobio.
2021
Gao C, Wisniewski L, Liu Y, Staal B, Beddows I, Plenker D, Aldakkak M, Hall J, Barnett D, Gouda MK, Allen P, Drake R, Zureikat A, Huang Y, Evans D, Singhi A, Brand RE, Tuveson DA, Tsai S, Haab BB. 2021. Detection of chemotherapy-resistant pancreatic cancer using a glycan biomarker, sTRA. Clin Cancer Res 27(1):226-236.
Klamer Z, Haab BB. 2021. Combined analysis of multiple glycan-array datasets: New explorations of protein-glycan interactions. Analyt Chem.
2020
McDowell CT, Klamer Z, Hall J, West CA, Wisniewski L, Powers TW, Angel P, Mehta AS, Lewin DN, Haab BB, Drake R. 2020. Imaging mass spectrometry and lectin analysis of N-linked glycans in carbohydrate antigen defined pancreatic cancer tissues. Mol Cell Proteomics 20:100012.
*Highlighted by ASBMBToday
Gao CF, Wisniewski LB, Liu Y, Staal B, Beddows I, Plenker D, Aldakkak M, Hall J, Barnett D, Kheir Gouda M, Allen PJ, Drake RR, Zureikat AM, Huang Y, Evans DB, Singhi AD, Brand RE, Tuveson DA, Tsai S, Haab BB. 2020. Detection of chemotherapy-resistant pancreatic cancer using a glycan biomarker, sTRA. Clin Cancer Res.
2019
Staal B*, Liu Y*, Barnett D*, Hsueh P, Zonglin H, Gao C, Partyka K, Hurd MW, Singhi AD, Drake RR, Huang Y, Maitra A, Brand RE, Haab BB. 2019. The sTRA plasma biomarker: Blinded validation of improved accuracy over CA19-9 in pancreatic cancer diagnosis. Clin Cancer Res.
Barnett D, Haab BB. 2019. Automated identification and quantification of signals in multichannel immunofluorescence images: The SignalFinder platform. Am J Pathol.
Klamer Z, Hsueh P, Ayala-Talavera D, Haab B. 2019. Deciphering protein glycosylation by computational integration of on-chip profiling, glycan-array data, and mass spectrometry. Mol Cell Proteomics 18(1):28-40.
2017
Klamer Z, Staal B, Prudden AR, Liu L, Smith DF, Boons GJ, Haab BB. 2017. Mining high-complexity motifs in glycans: A new language to uncover the fine specificities of lectins and glycosidases.
Anal Chem.
Barnett D, Liu Y, Partyka K, Huang Y, Tang H, Hostetter G, Brand RE, Singhi AD, Drake RR, Haab BB. 2017. The CA19-9 and Sialyl-TRA antigens define separate subpopulations of pancreatic cancer cells. Sci Rep 7:4020.
2016
Reatini BS, Ensink E, Liau B, Sinha JY, Powers TW, Partyka K, Bern M, Brand RE, Rudd PM, Kletter D, Drake RR, Haab BB. 2016. Characterizing protein glycosylation through on-chip glycan medication and probing. Anal Chem.
Tang H, Partyka K, Hsueh P, Sinha JY, Kletter D, Zeh H, Huang Y, Brand RE, Haab BB. 2016. Glycans related to the CA19-9 antigen are elevated in distinct subsets of pancreatic cancers and improve diagnostic accuracy over CA19-9. Cell Mol Gastroenterol Hepatol 2(2):201–215.
2015
Haab BB, Huang Y, Balasenthil S, Partyka K, Tang H, Anderson M, Allen P, Sasson A, Zeh H, Kaul K, Kletter D, Ge S, Bern M, Kwon R, Blasutig I, Srivastava S, Frazier ML, Sen S, Hollingsworth MA, Rinaudo JA, Killary AM, Brand RE. 2015. Definitive characterization of CA 19-9 in resectable pancreatic cancer using a reference set of serum and plasma specimens. PLoS One. 10(10):e0139049.
Ensink E, Sinha J, Sinha A, Tang H, Calderone HM, Hostetter G, Winter J, Cherba D, Brand RE, Allen PJ, Sempere LF, Haab BB. 2015. Segment and fit thresholding: A new method for image analysis applied to microarray and immunofluorescence data. Anal Chem 87(19):9715–9721.
Tang H, Hsueh P, Kletter D, Bern M, Haab BB. 2015. The detection and discovery of glycan motifs in biological samples using lectins and antibodies: new methods and opportunities. Adv Cancer Res 126:167–202.
Kletter D, Curnutte B, Maupin K, Bern M, Haab BB. 2015. Exploring the specificities of glycan-binding proteins using glycan array data and the GlycoSearch software. Methods Mol Biol 1273:203–214.
Tang H, Singh S, Partyka K, Kletter D, Hsueh P, Yadav J, Ensink E, Bern M, Hostetter G, Hartman D, Huang Y, Brand RE, Haab BB. 2015. Glycan motif profiling reveals plasma sialyl-Lewis X elevations in pancreatic cancers that are negative for CA 19-9. Mol Cell Proteomics 14(5):1323–1333.
Singh S, Pal K, Yadav J, Tang H, Partyka K, Kletter D, Hsueh P, Ensink E, Birenda KC, Hostetter G, Xu H, Bern M, Smith D, Mehta A, Brand R, Melcher K, Haab BB. 2015. Upregulation of glycans containing 31 fucose in a subset of pancreatic cancers uncovered using fusion-tagged lectins. J Proteome Res 14(6):2594–605.
2014
Powers TW, Neely BA, Shao Y, Tang H, Troyer DA, Mehta AS, Haab BB, Drake RR. MALDI imaging mass spectrometry profiling of N-glycans in formalin-fixed paraffin embedded clinical tissue blocks and tissue microarrays. PLoS One 9(9):e106255.
Kletter D, Bern M, Haab BB. 2014. Mining and using glycan array data with the GlycoSearch analysis program and GlycanBinder database. In: Naoyuki Taniguchi, T. E., Gerald Hart, Peter Seeberger, Chi-Huey Wong, ed. Glycoscience: Biology and Medicine, Springer, Japan.
Sinha A, Cherba D, Bartlam H, Lenkiewicz E, Evers L, Barrett MT, Haab BB. 2014. Mesenchymal-like pancreatic cancer cells harbor specific genomic alterations more frequently than their epithelial counterparts. Mol Oncol 8(7):1253–1265.
Partyka K, Wang S, Zhao P, Haab BB. 2014. Array-based immunoassays with rolling-circle amplification detection. Methods Mol Biol 1105: 3–15.
Gbormittah FO, Haab BB, Partyka K, Garcia-Ott C, Hincapie M, Hancock WS. 2014. Characterization of glycoproteins in pancreatic cyst fluid using a high performance multiple lectin affinity chromatography platform. J Proteome Res 13(1): 289–299.
2013
Cao Z, Maupin K, Curnutte B, Fallon B, Feasley CL, Brouhard E, Kwon R, West CM, Cunningham J, Brand R, Castelli P, Crippa S, Feng Z, Allen P, Simeone DM, Haab BB. 2013. Specific glycoforms of MUC5AC and endorepellin accurately distinguish mucinous from non-mucinous pancreatic cysts. Mol Cell Proteomics 12(10): 2724–2734.
McCarter C, Kletter D, Tang H, Partyka K, Ma Y, Singh S, Yadav J, Bern M, Haab BB. 2013. Prediction of glycan motifs using quantitative analysis of multi-lectin binding: motifs on MUC1 produced by cultured pancreatic cancer cells. Proteomics Clin Appl 7(9–10): 632–641.
Kletter D, Cao Z, Bern M, Haab BB. 2013. Determining lectin specificity from glycan array data using motif segregation and the GlycoSearch software. Curr Protoc Chem Biol 5(2): 157–169.
Haab BB, Partyka K, Cao Z. 2013. Using antibody arrays to measure protein abundance and glycosylation: considerations for optimal performance. Curr Protoc Protein Sci 73: Unit 27.6.
Fallon BP, Curnutte B, Maupin KA, Partyka K, Choi S, Brand RE, Langmead CJ, Tembe W, Haab BB. 2013. The Marker State Space (MSS) method for classifying clinical samples. PLoS One 8(6):e65905.
Kletter D, Singh S, Bern M, Haab BB. 2013. Global comparisons of lectin-glycan interactions using a database of analyzed glycan array data. Molecular & Cellular Proteomics 12(4):1026–1035.
Cao Z, Partyka K, McDonald M, Brouhard E, Hincapie M, Brand RE, Hancock WS, Haab BB. 2013. Modulation of glycan detection on specific glycoproteins by lectin multimerization. Anal Chem 85(3):1689–1698.
2012
Haab BB. 2012. Using lectins in biomarker research: addressing the limitations of sensitivity and availability. Proteomics Clin Appl 6(7-8):346–350.
Maupin KA, Liden D, Haab BB. 2012. The fine-specificity of mannose-binding and galactose-binding lectins revealed using outlier-motif analysis of glycan array data. Glycobiology 22(1):160–169.
Partyka K, Maupin KA, Brand RE, Haab BB. 2012. Diverse monoclonal antibodies against the CA 19-9 antigen show variation in binding specificity with consequences for clinical interpretation. Proteomics 12(13): 2212–2220.
Partyka K, McDonald M, Maupin KA, Brand R, Kwon R, Simeone DM, Allen P, Haab BB. 2012. Comparison of surgical and endoscopic sample collection for pancreatic cyst fluid biomarker identification. J Proteome Res 11(5): 2904–2911.
2011
Yue T, Maupin K, Fallon B, Li L, Partyka K, Anderson M, Brenner DE, Kaul K, Zeh H, Moser AJ, Simeone DM, Feng Z, Brand RE, Haab BB. 2011. Enhanced discrimination of malignant from benign pancreatic disease by measuring the CA 19-9 antigen on specific protein carriers. PLoS One 6(12): e29180.
Yue T, Partyka K, Maupin K, Hurley M, Andrews P, Kaul K, Moser JA, Zeh H, Brand RE, Haab BB. 2011. Identification of blood-protein carriers of the CA 19-9 antigen and characterization of prevalence in pancreatic diseases. Proteomics 11(18):3665–3674.
2010
Bergsma D, Buchweitz J, Chen S, Gerszten R, Haab BB. 2010. Antibody-array interaction mapping, a new method to detect protein complexes applied to the discovery and study of serum amyloid P interactions with kininogen in human plasma. Mol Cell Proteomics 9(3): 446–456.
Haab BB. 2010. Antibody-lectin sandwich arrays for biomarker and glycobiology studies. Expert Rev Proteomics 7(1): 9–11.
Haab BB, Porter A, Yue T, Li L, Scheiman J, Anderson MA, Barnes D, Schmidt CM, Feng Z, Simeone DM. 2010. Glycosylation variants of mucins and CEACAMs as candidate biomarkers for the diagnosis of pancreatic cystic neoplasms. Ann Surg 251(5): 937–945.
Maupin KA, Sinha A, Eugster E, Miller J, Ross J, Paulino V, Keshamouni VG, Tran N, Berens M, Webb C, Haab BB. 2010. Glycogene expression alterations associated with pancreatic cancer epithelial-mesenchymal transition in complementary model systems. PLoS One 5(9): e13002.
Porter A, Yue T, Heeringa L, Day S, Suh E, Haab BB. 2010. A motif-based analysis of glycan array data to determine the specificities of glycan-binding proteins. Glycobiology 20(3): 369–380.
Zeng Z, Hincapie M, Haab BB, Hanash S, Pitteri SJ, Kluck S, Hogan JM, Kennedy J, Hancock WS. 2010. The development of an integrated platform to identify breast cancer glycoproteome changes in human serum. J Chromatogr A 1217(19): 3307–3315.
2009
Hung KE, Faca V, Song K, Sarracino DA, Richard LG, Krastins B, Forrester S, Porter A, Kunin A, Mahmood U, Haab BB, Hanash SM, Kucherlapati R. 2009. Comprehensive proteome analysis of an Apc mouse model uncovers proteins associated with intestinal tumorigenesis. Cancer Prev Res (Phila) 2(3): 224–233.
Shao C, Chen S, Chen L, Cobos E, Wang JS, Haab BB, Gao W. 2009. Antibody microarray analysis of serum glycans in esophageal squamous cell carcinoma cases and controls. Proteomics Clin Appl 3(8): 923–931.
Wu YM, Nowack DD, Omenn GS, Haab BB. 2009. Mucin glycosylation is altered by pro-inflammatory signaling in pancreatic cancer cells. J Proteome Res 8(4):1876–1886.
Yue T, Goldstein IJ, Hollingsworth MA, Kaul K, Brand RE, Haab BB. 2009. The prevalence and nature of glycan alterations on specific proteins in pancreatic cancer patients revealed using antibody-lectin sandwich arrays. Mol Cell Proteomics 8(7): 1697–1707.
2007
Chen S, LaRoche T, Hamelinck D, Bergsma D, Brenner D, Simeone D, Brand RE, Haab BB. 2007. Multiplexed analysis of glycan variation on native proteins captured by antibody microarrays. Nat Methods 4(5): 437–444.
Forrester S, Hung KE, Kuick R, Kucherlapati R, Haab BB. 2007. Low-volume, high-throughput sandwich immunoassays for profiling plasma proteins in mice: identification of early-stage systemic inflammation in a mouse model of intestinal cancer. Mol Oncol 1(2): 216–225.
Forrester S, Qiu J, Mangold L, Partin A, Misek D, Phinney B, Whitten D, Andrews P, Diamandis D, Omenn GS, Hanash S, Haab BB. 2007. Multiplexed analysis of glycan variation on native proteins captured by antibody microarrays. Proteomics Clin Appl 1(5): 494–505.
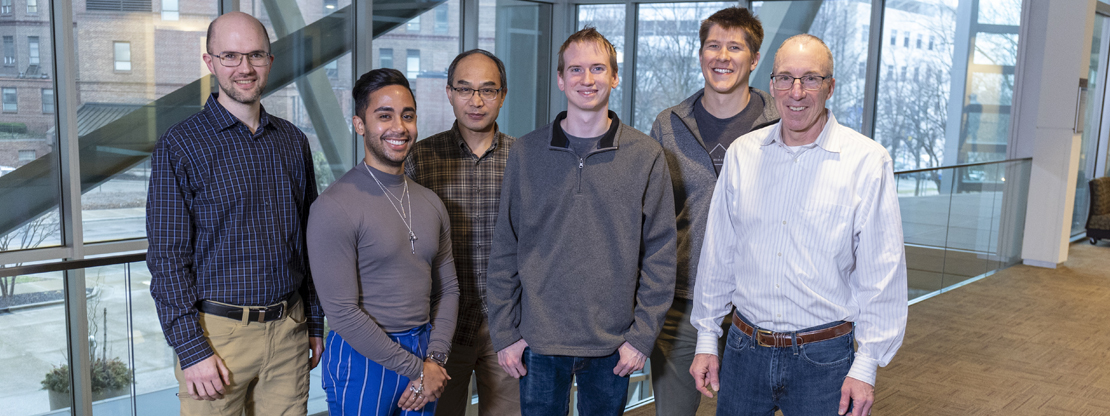
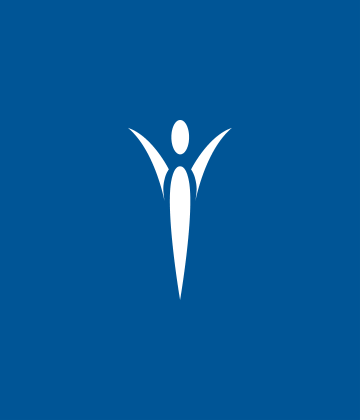
Braelyn Binkowski
Assistant Research Technician, Department of Cell Biology

Wendy Fritz
Senior Administrative Assistant I, Department of Cell Biology
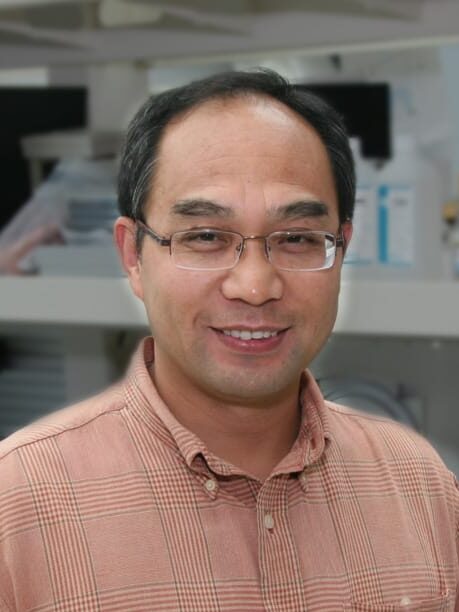
ChongFeng Gao, Ph.D.
Senior Research Scientist, Department of Cell Biology
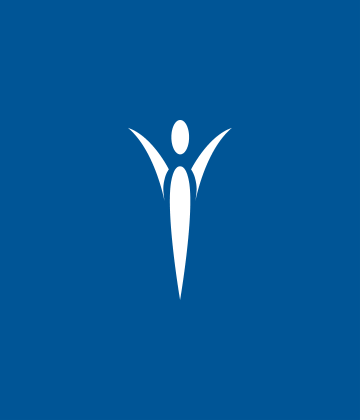
Zachary Klamer, M.S.
Bioinformatics Analyst II, Department of Cell Biology
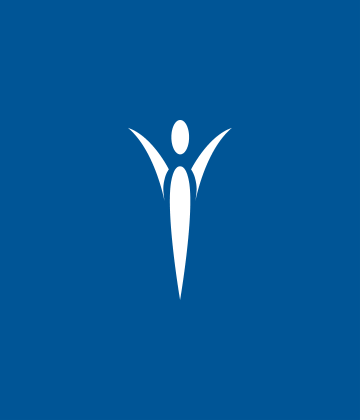
Gabrielle Lathrop
Student Intern
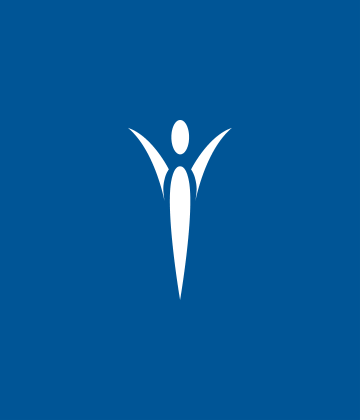
Anna Rapesh, M.S.
Bioinformatician I, Department of Cell Biology

Alfredo Reyes Oliveras
Ph.D. Candidate, VAI Graduate School
Thesis: Linking glycosylation changes in pancreatic cancer with metabolic dependencies
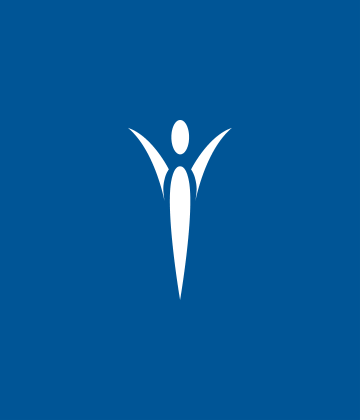
Ben Staal, M.S.
Research Associate, Department of Cell Biology
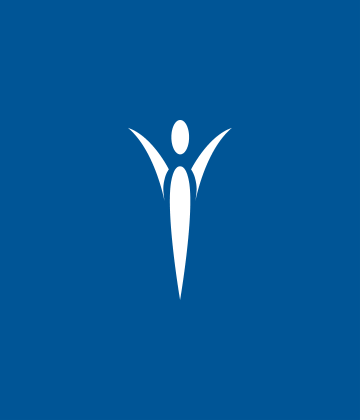
Hoang-Le Tran, M.S.
Bioinformatics Analyst I, Department of Cell Biology